Semantic taxonomy induction
Semantic taxonomy induction(2009)
摘要
Understanding natural language has been a longstanding dream of artificial intelligence, and machine learning offers a new perspective on this old problem. This work addresses four key problems in automatically reading and understanding text: extracting the knowledge expressed in a body of text in the form of structured relations, reconciling and formalizing that knowledge in a fully consistent, sense-disambiguated hierarchy of knowledge, fluidly transitioning from fine-grained to coarse-grained distinctions between word senses, and applying extracted structured knowledge in applications that depend on deep textual understanding. Textual patterns have frequently been devised to identify specific instances of world knowledge in text. For example, from the text “such fruits as apples and oranges” one might infer the knowledge that “apples and oranges are kinds of fruit”. In this work we discuss the use of distant supervision for relation extraction, which applies machine learning techniques to a set of example relation instances and a large body of unannotated text in order to rediscover many of the textual patterns formerly proposed in the information extraction literature, along with hundreds of thousands of previously unconsidered patterns. Further, we apply these automatically discovered patterns to extract structured knowledge from newswire articles and other text, significantly outperforming hand-designed patterns and discovering hundreds of thousands of novel examples of world knowledge not previously encoded in manually-created knowledge bases. Many proposed methods for extracting structured knowledge suffer from a critical inability to deal with redundancy or contradictory extractions. While modern algorithms can often suggest millions of possible facts extracted from a large body of text, they are unable to reconcile this extracted knowledge into a set of consistent, sense-disambiguated assertions. We propose a probabilistic framework for taxonomy induction that solves each of these problems, taking advantage of the full set of predicted facts and any knowledge already known in an existing taxonomy. This work has resulted in one of the largest automatically-constructed augmentations of the WordNet knowledge base currently in existence. In addition to the automatic augmentation of knowledge resources, we explore the task of automatically creating coarse-grained taxonomies. It has been widely observed that different natural language applications require different sense granularities in order to best exploit word sense distinctions, and that for many applications WordNet senses are too fine-grained. In contrast to previously proposed automatic methods for sense clustering, we formulate sense merging as a supervised learning problem, exploiting human-labeled sense clusterings as training data. Our learned similarity measure outperforms previously proposed automatic methods for sense clustering on the task of predicting human sense merging judgments. Finally, we propose a model for clustering sense taxonomies using the outputs of this classifier, and we make available several automatically sense-clustered WordNets of various sense granularities. These resources offer the capability of tailoring a knowledge resource to the sense granularity most suited to a particular application. Our framework for taxonomy induction lays the groundwork for new semantic applications, including inferring domain-specific hierarchies of knowledge and augmenting foreign-language Wordnets. Finally, we demonstrate that our automatically augmented taxonomies significantly outperform manually-constructed resources across several natural language tasks, including relation prediction, question answering, and text categorization.
更多查看译文
关键词
different sense granularity,world knowledge,WordNet knowledge base,human-labeled sense clusterings,structured knowledge,clustering sense,applications WordNet sense,manually-created knowledge base,human sense,knowledge resource,semantic taxonomy induction
AI 理解论文
溯源树
样例
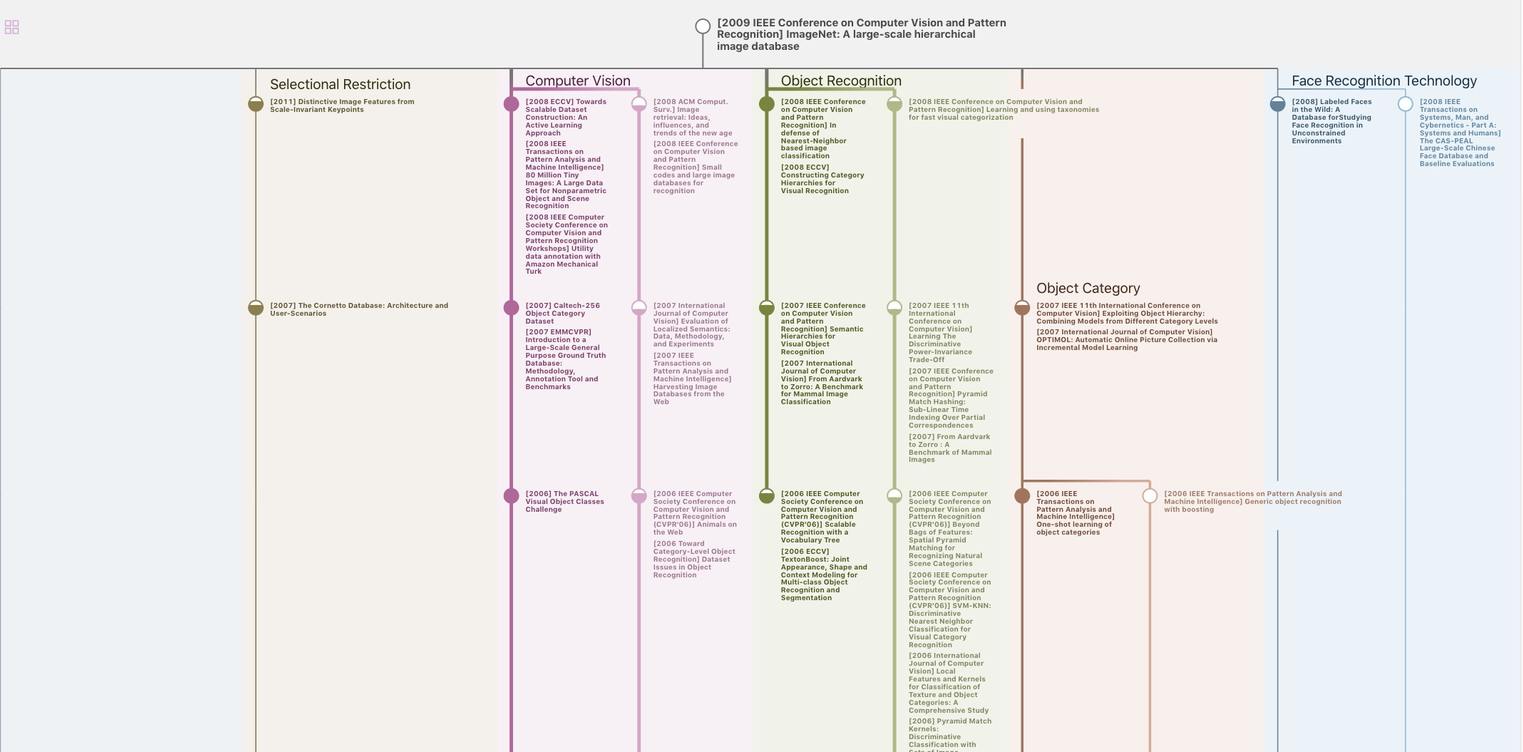
生成溯源树,研究论文发展脉络
Chat Paper
正在生成论文摘要