Active Risk Estimation
ICML(2010)
摘要
We address the problem of evaluating the risk of a given model accurately at minimal la- beling costs. This problem occurs in situa- tions in which risk estimates cannot be ob- tained from held-out training data, because the training data are unavailable or do not re- ect the desired test distribution. We study active risk estimation processes in which in- stances are actively selected by a sampling process from a pool of unlabeled test in- stances and their labels are queried. We de- rive the sampling distribution that minimizes the estimation error of the active risk esti- mator when used to select instances from the pool. An analysis of the distribution that governs the estimator leads to condence in- tervals. We empirically study conditions un- der which the active risk estimate is more accurate than a standard risk estimate that draws equally many instances from the test distribution.
更多查看译文
关键词
empirical study
AI 理解论文
溯源树
样例
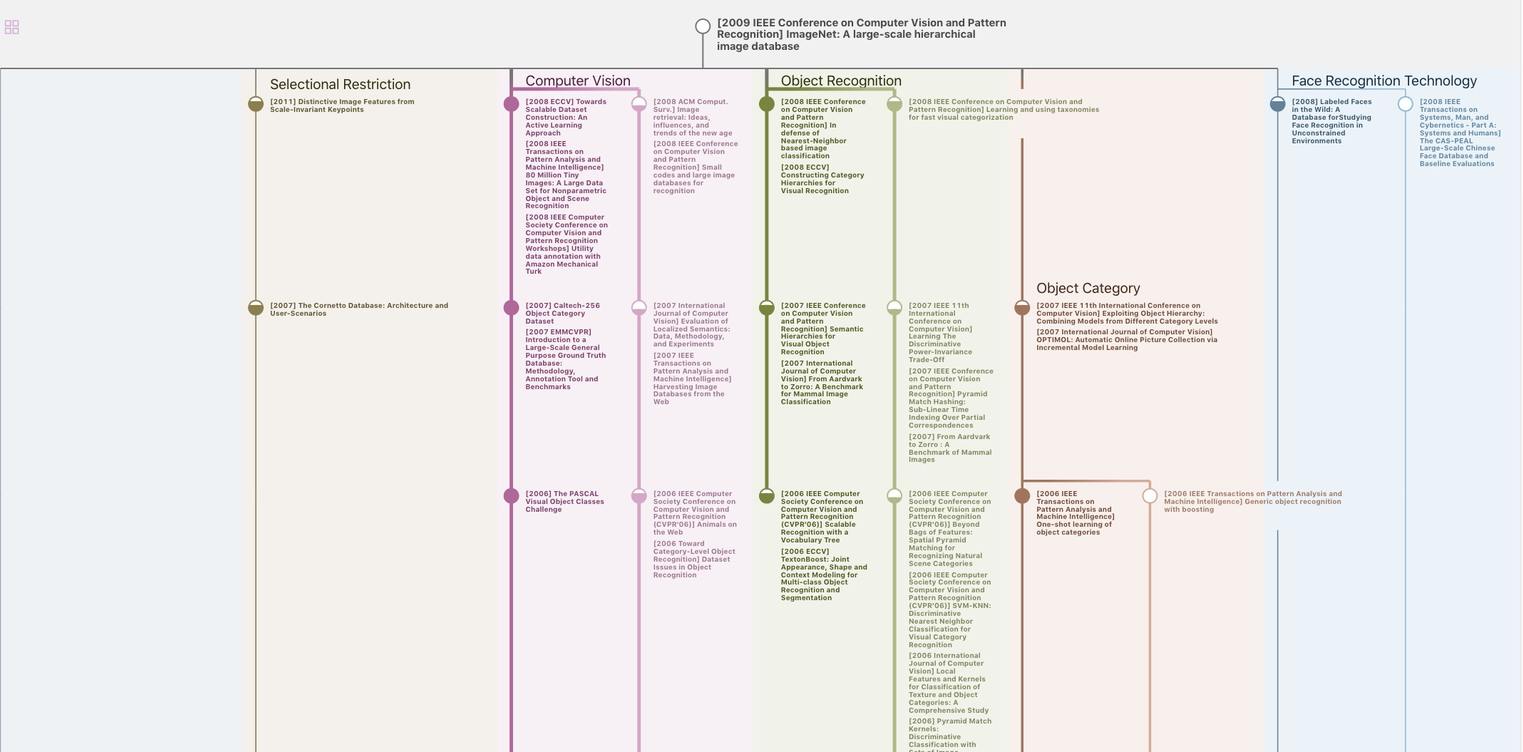
生成溯源树,研究论文发展脉络
Chat Paper
正在生成论文摘要