Sample Propagation
NIPS(2003)
摘要
Rao-Blackwellization is an approximation technique for probabilistic in- ference that flexibly combines exact inference with sampling. It is useful in models where conditioning on some of the variables leaves a sim- pler inference problem that can be solved tractably. This paper presents Sample Propagation, an efficient implementation of Rao-Blackwellized approximate inference for a large class of models. Sample Propagation tightly integrates sampling with message passing in a junction tree, and is named for its simple, appealing structure: it walks the clusters of a junction tree, sampling some of the current cluster's variables and then passing a message to one of its neighbors. We discuss the application of Sample Propagation to conditional Gaussian inference problems such as switching linear dynamical systems.
更多查看译文
AI 理解论文
溯源树
样例
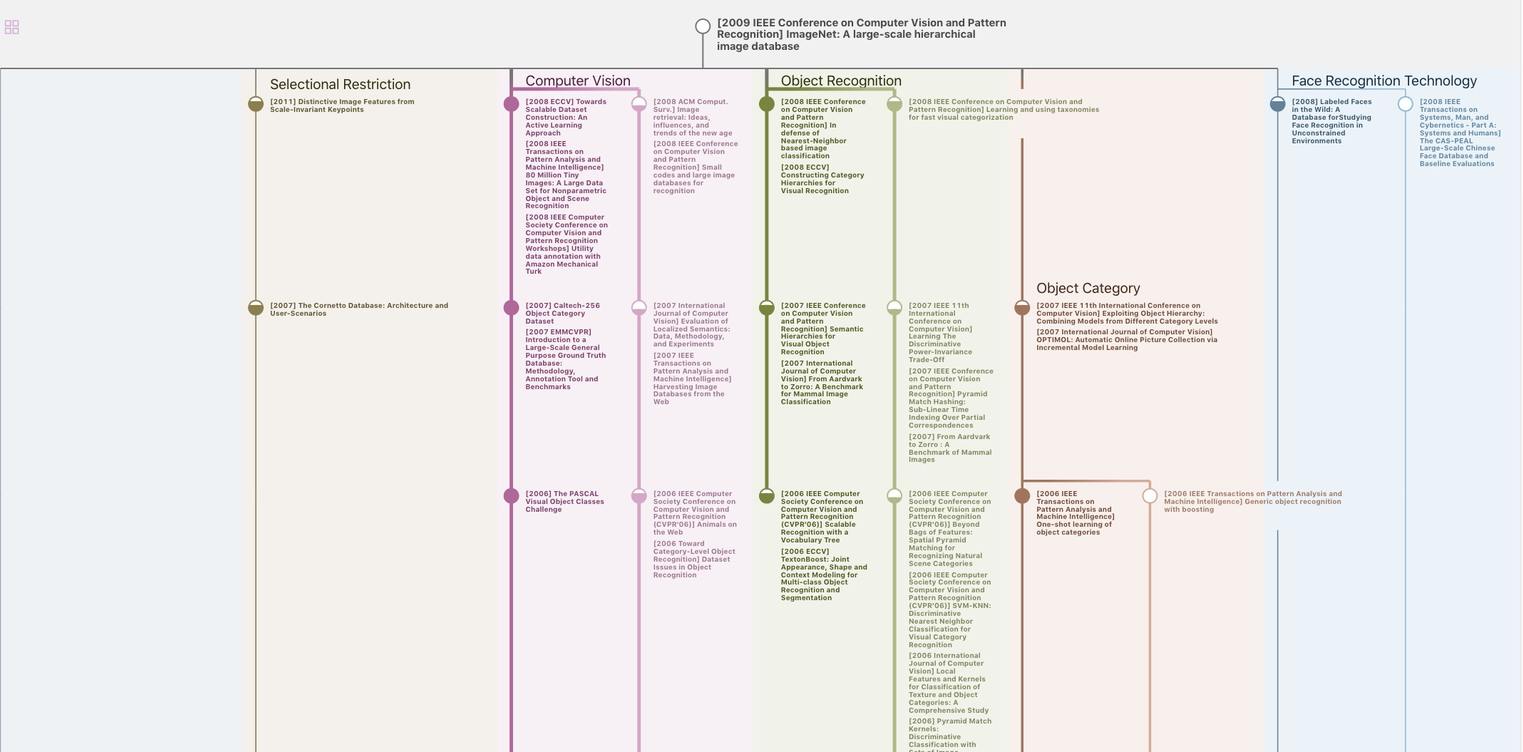
生成溯源树,研究论文发展脉络
Chat Paper
正在生成论文摘要