Probabilistic speech feature extraction with context-sensitive Bottleneck neural networks
Neurocomputing(2014)
摘要
We introduce a novel context-sensitive feature extraction approach for spontaneous speech recognition. As bidirectional Long Short-Term Memory (BLSTM) networks are known to enable improved phoneme recognition accuracies by incorporating long-range contextual information into speech decoding, we integrate the BLSTM principle into a Tandem front-end for probabilistic feature extraction. Unlike the previously proposed approaches which exploit BLSTM modeling by generating a discrete phoneme prediction feature, our feature extractor merges continuous high-level probabilistic BLSTM features with low-level features. By combining BLSTM modeling and Bottleneck (BN) feature generation, we propose a novel front-end that allows us to produce context-sensitive probabilistic feature vectors of arbitrary size, independent of the network training targets. Evaluations on challenging spontaneous, conversational speech recognition tasks show that this concept prevails over recently published architectures for feature-level context modeling.
更多查看译文
关键词
blstm principle,blstm modeling,feature generation,discrete phoneme prediction feature,context-sensitive bottleneck neural network,probabilistic feature extraction,probabilistic speech feature extraction,continuous high-level probabilistic blstm,context-sensitive probabilistic feature vector,novel context-sensitive feature extraction,low-level feature,feature extractor,long short term memory
AI 理解论文
溯源树
样例
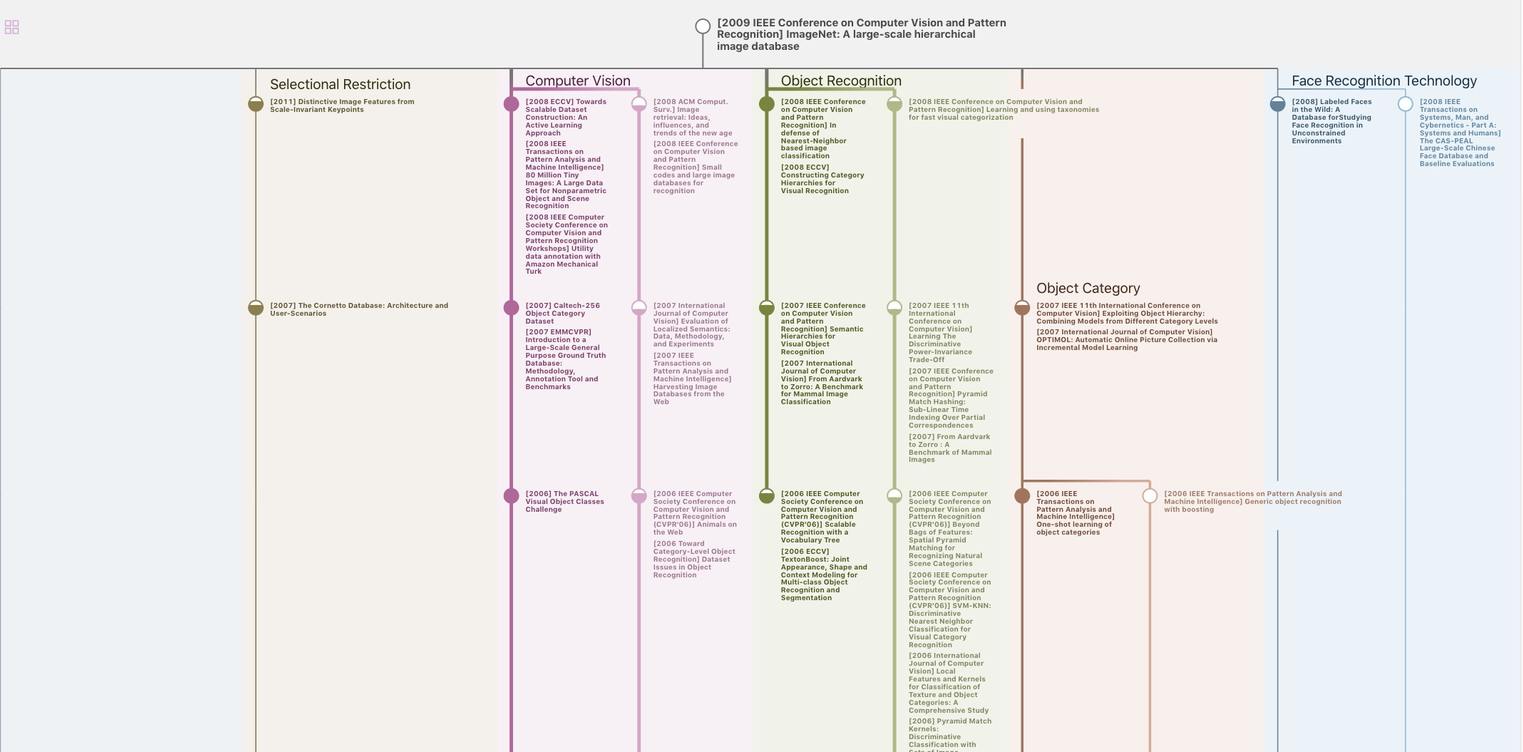
生成溯源树,研究论文发展脉络
Chat Paper
正在生成论文摘要