Alternating Regression Forests for Object Detection and Pose Estimation
Computer Vision(2013)
摘要
We present Alternating Regression Forests (ARFs), a novel regression algorithm that learns a Random Forest by optimizing a global loss function over all trees. This interrelates the information of single trees during the training phase and results in more accurate predictions. ARFs can minimize any differentiable regression loss without sacrificing the appealing properties of Random Forests, like low computational complexity during both, training and testing. Inspired by recent developments for classification [19], we derive a new algorithm capable of dealing with different regression loss functions, discuss its properties and investigate the relations to other methods like Boosted Trees. We evaluate ARFs on standard machine learning benchmarks, where we observe better generalization power compared to both standard Random Forests and Boosted Trees. Moreover, we apply the proposed regressor to two computer vision applications: object detection and head pose estimation from depth images. ARFs outperform the Random Forest baselines in both tasks, illustrating the importance of optimizing a common loss function for all trees.
更多查看译文
关键词
object detection,different regression loss function,random forest baselines,global loss function,boosted trees,standard random forests,alternating regression forests,differentiable regression loss,random forests,common loss function,pose estimation,random forest,regression forests,regression analysis,learning artificial intelligence,computational complexity,image classification,computer vision
AI 理解论文
溯源树
样例
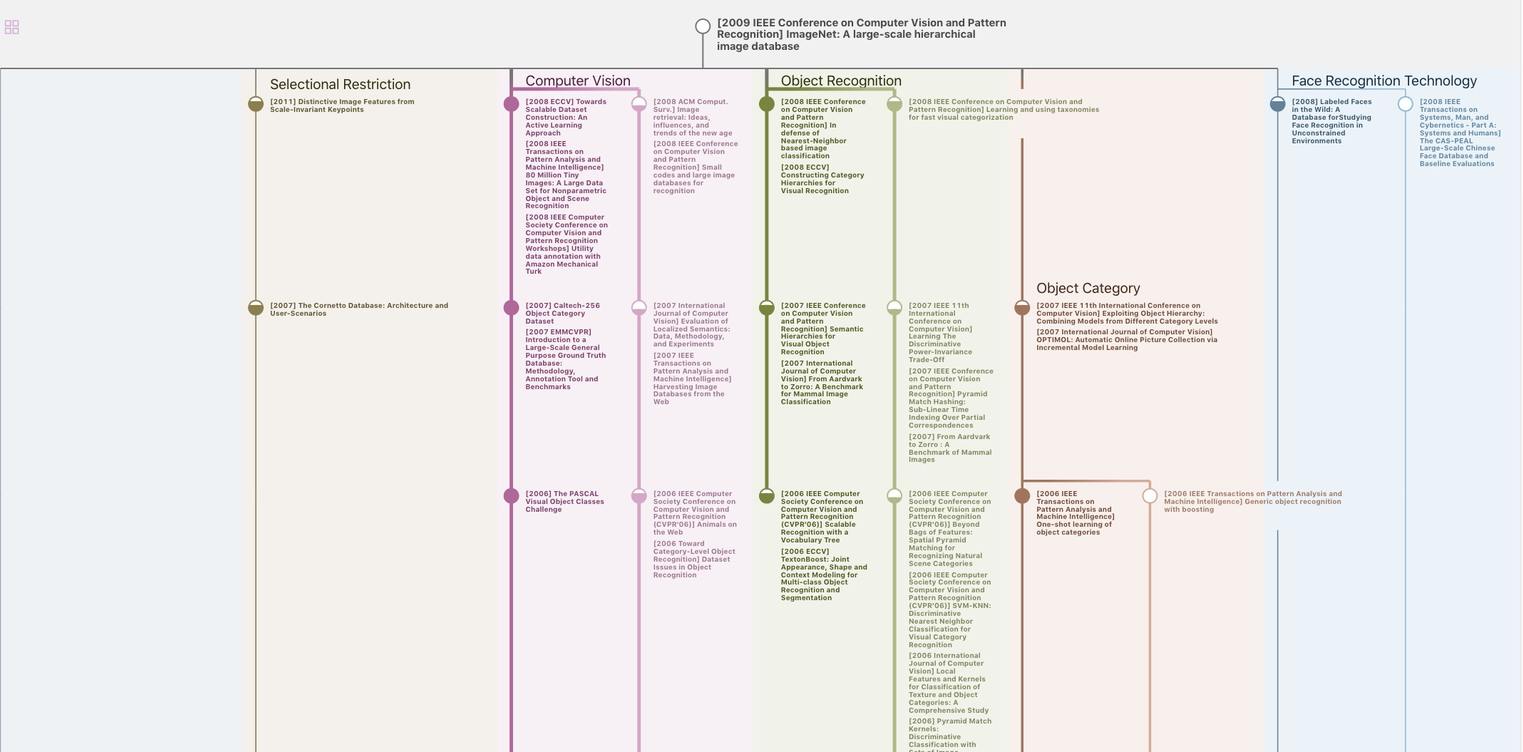
生成溯源树,研究论文发展脉络
Chat Paper
正在生成论文摘要