Codemaps - Segment, Classify and Search Objects Locally
Computer Vision(2013)
摘要
In this paper we aim for segmentation and classification of objects. We propose codemaps that are a joint formulation of the classification score and the local neighborhood it belongs to in the image. We obtain the codemap by reordering the encoding, pooling and classification steps over lattice elements. Other than existing linear decompositions who emphasize only the efficiency benefits for localized search, we make three novel contributions. As a preliminary, we provide a theoretical generalization of the sufficient mathematical conditions under which image encodings and classification becomes locally decomposable. As first novelty we introduce l2 normalization for arbitrarily shaped image regions, which is fast enough for semantic segmentation using our Fisher codemaps. Second, using the same lattice across images, we propose kernel pooling which embeds nonlinearities into codemaps for object classification by explicit or approximate feature mappings. Results demonstrate that l2 normalized Fisher codemaps improve the state-of-the-art in semantic segmentation for PASCAL VOC. For object classification the addition of nonlinearities brings us on par with the state-of-the-art, but is 3x faster. Because of the codemaps' inherent efficiency, we can reach significant speed-ups for localized search as well. We exploit the efficiency gain for our third novelty: object seg- ment retrieval using a single query image only.
更多查看译文
关键词
fisher codemaps,image encodings,classification step,semantic segmentation,object classification,classification score,normalized fisher codemaps,localized search,shaped image region,single query image,image classification,image segmentation,image retrieval
AI 理解论文
溯源树
样例
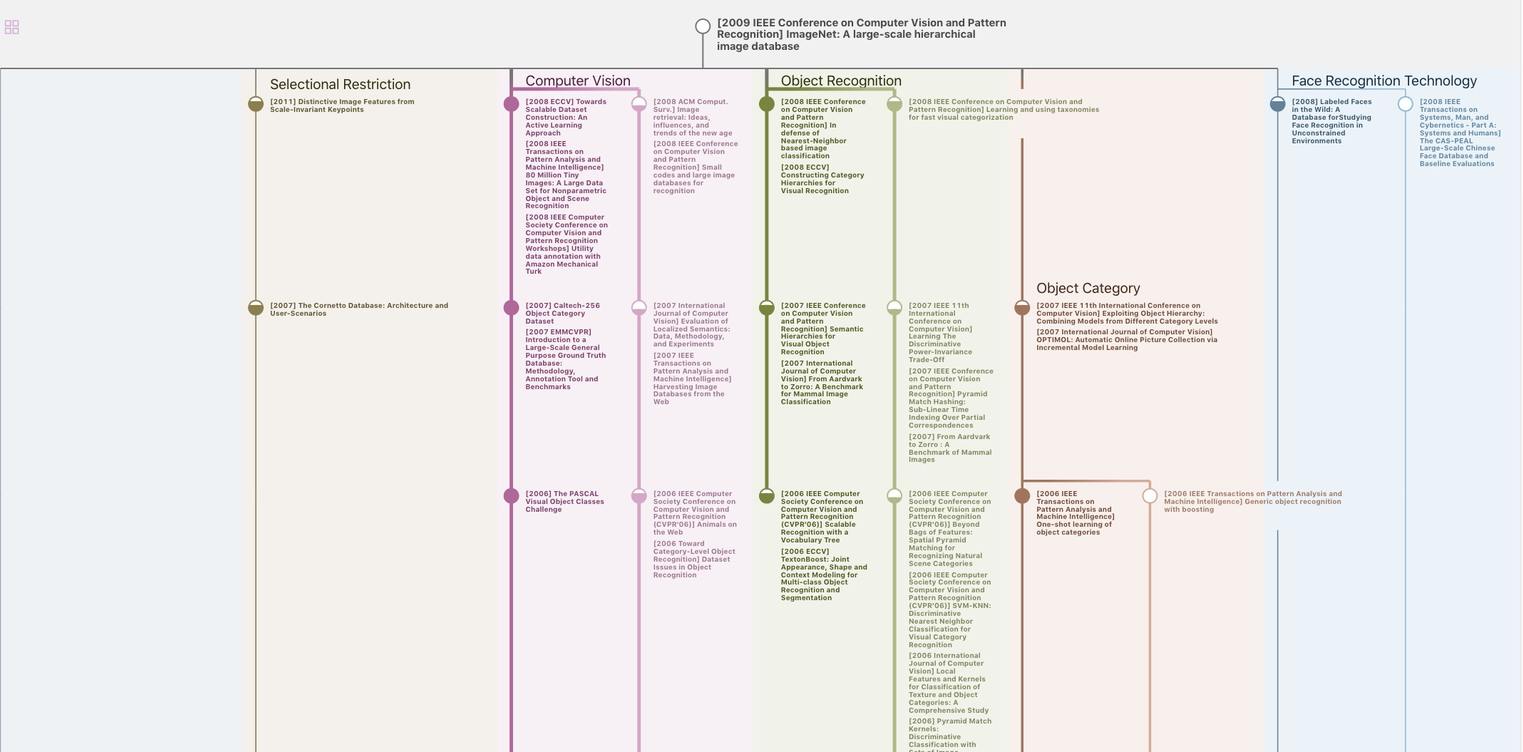
生成溯源树,研究论文发展脉络
Chat Paper
正在生成论文摘要