On the construction of the relevance vector machine based on Bayesian Ying-Yang harmony learning.
Neural Networks(2013)
摘要
Tipping's relevance vector machine (RVM) applies kernel methods to construct basis function networks using a least number of relevant basis functions. Compared to the well-known support vector machine (SVM), the RVM provides a better sparsity, and an automatic estimation of hyperparameters. However, the performance of the original RVM purely depends on the smoothness of the presumed prior of the connection weights and parameters. Consequently, the sparsity is actually still controlled by the choice of kernel functions and/or kernel parameters. This may lead to severe underfitting or overfitting in some cases. In this research, we explicitly involve the number of basis functions into the objective of the optimization procedure, and construct the RVM by maximizing the harmony function between "hypothetical" probability distribution in the forward training pathway and "true" probability distribution in the backward testing pathway, using Xu's Bayesian Ying-Yang (BYY) harmony learning technique. The experimental results have shown that our proposed methodology can achieve both the least complexity of structure and goodness of fit to data.
更多查看译文
关键词
kernel parameter,bayesian inference,bayesian ying-yang harmony learning,bayesian ying-yang (byy) harmony learning,original rvm,relevant basis function,basis function,relevance vector machine,basis function network,probability distribution,forward training pathway,kernel method,relevance vector machine (rvm),better sparsity,kernel function
AI 理解论文
溯源树
样例
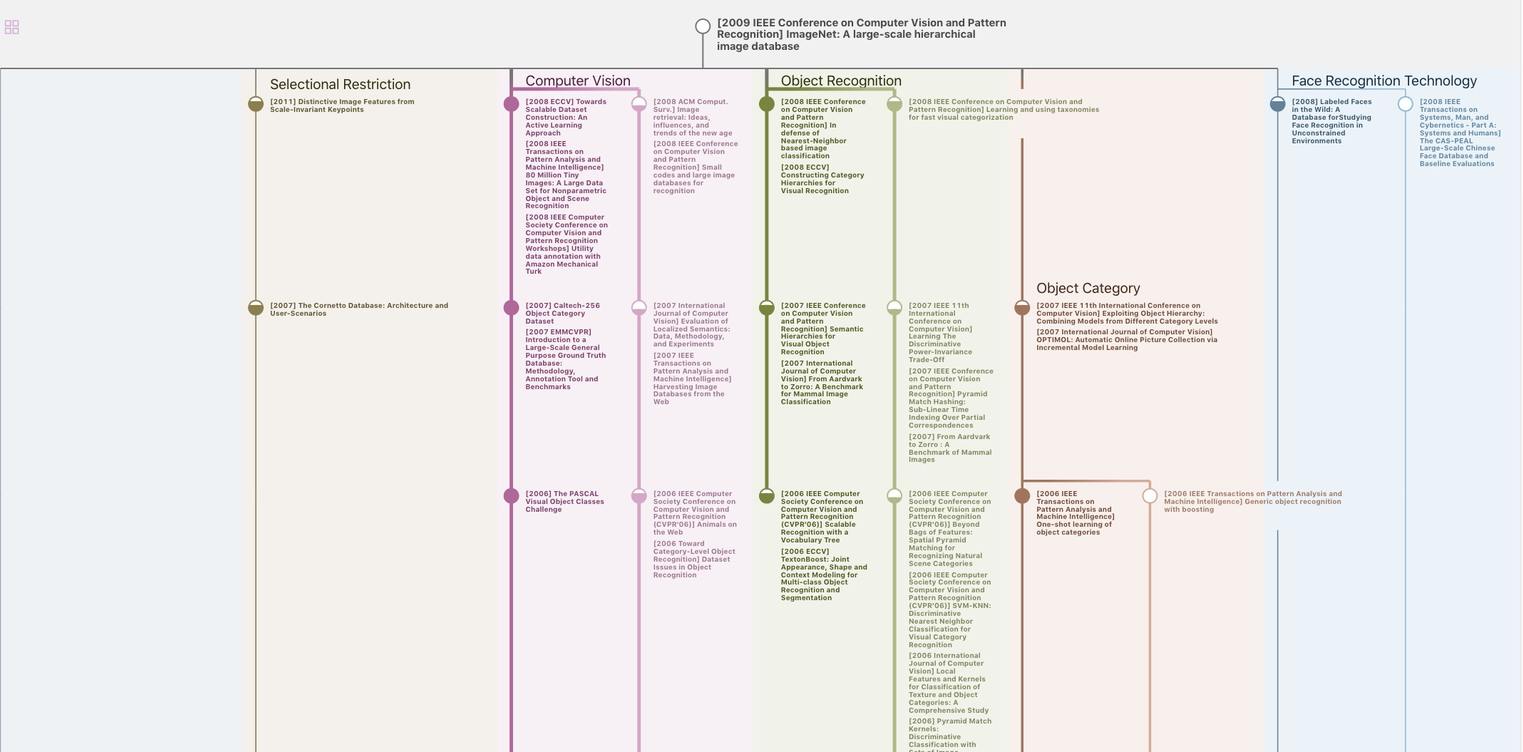
生成溯源树,研究论文发展脉络
Chat Paper
正在生成论文摘要