RASL: Robust Alignment by Sparse and Low-Rank Decomposition for Linearly Correlated Images
IEEE Transactions on Pattern Analysis and Machine Intelligence(2012)
摘要
This paper studies the problem of simultaneously aligning a batch of linearly correlated images despite gross corruption (such as occlusion). Our method seeks an optimal set of image domain transformations such that the matrix of transformed images can be decomposed as the sum of a sparse matrix of errors and a low-rank matrix of recovered aligned images. We reduce this extremely challenging optimization problem to a sequence of convex programs that minimize the sum of \ell^1-norm and nuclear norm of the two component matrices, which can be efficiently solved by scalable convex optimization techniques. We verify the efficacy of the proposed robust alignment algorithm with extensive experiments on both controlled and uncontrolled real data, demonstrating higher accuracy and efficiency than existing methods over a wide range of realistic misalignments and corruptions.
更多查看译文
关键词
image domain,component matrix,robust alignment,linearly correlated images,sparse matrix,convex program,low-rank matrix,optimization problem,low-rank decomposition,extensive experiment,scalable convex optimization technique,higher accuracy,gross corruption,convex optimization,sparse decomposition,algorithm design and analysis,convex programming,sparse matrices,matrix decomposition,optimization,robustness,computer graphics,pixel,mathematical model,minimization,low rank matrix,image registration,lighting,automation,convergence
AI 理解论文
溯源树
样例
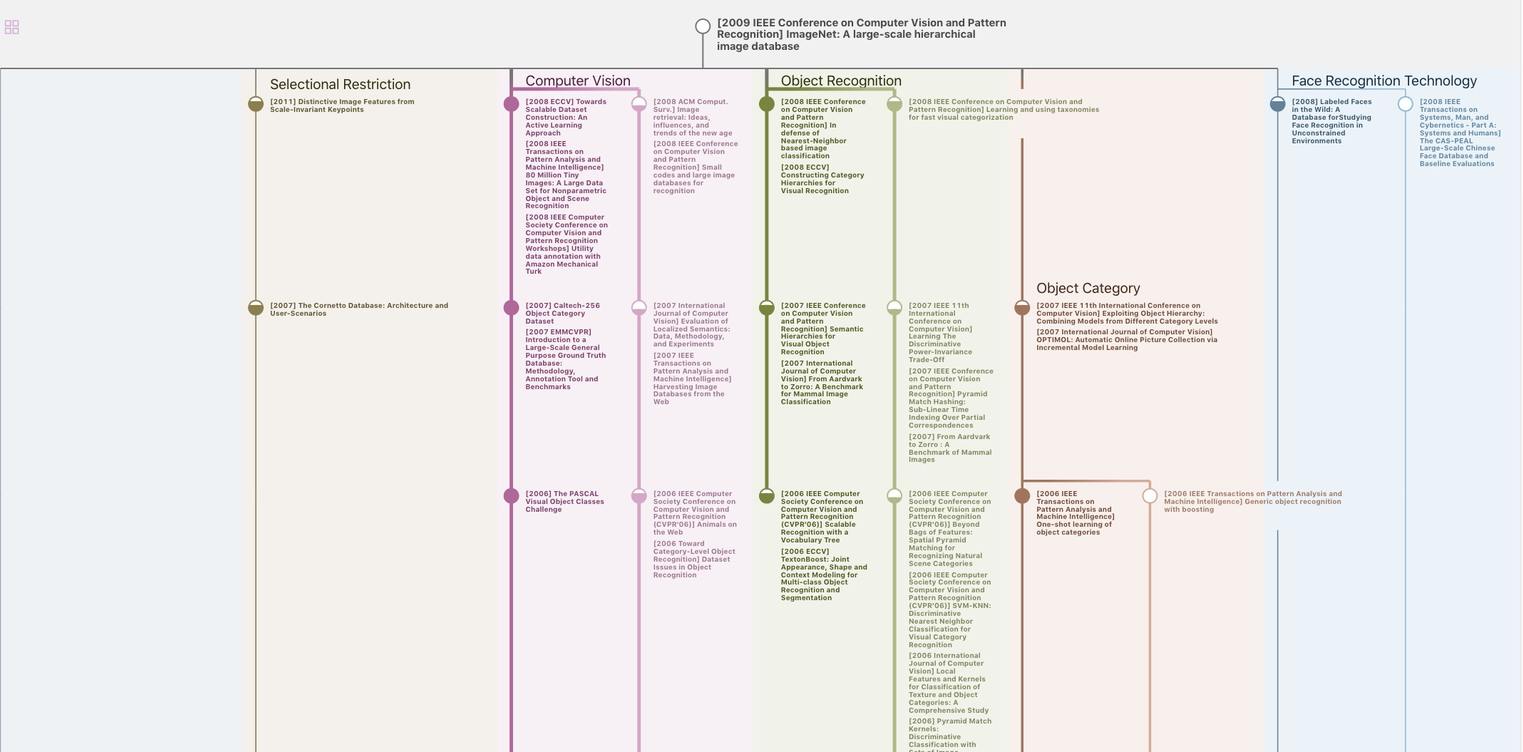
生成溯源树,研究论文发展脉络
Chat Paper
正在生成论文摘要