URL: A unified reinforcement learning approach for autonomic cloud management
Journal of Parallel and Distributed Computing(2012)
摘要
Cloud computing is emerging as an increasingly important service-oriented computing paradigm. Management is a key to providing accurate service availability and performance data, as well as enabling real-time provisioning that automatically provides the capacity needed to meet service demands. In this paper, we present a unified reinforcement learning approach, namely URL, to automate the configuration processes of virtualized machines and appliances running in the virtual machines. The approach lends itself to the application of real-time autoconfiguration of clouds. It also makes it possible to adapt the VM resource budget and appliance parameter settings to the cloud dynamics and the changing workload to provide service quality assurance. In particular, the approach has the flexibility to make a good trade-off between system-wide utilization objectives and appliance-specific SLA optimization goals. Experimental results on Xen VMs with various workloads demonstrate the effectiveness of the approach. It can drive the system into an optimal or near-optimal configuration setting in a few trial-and-error iterations.
更多查看译文
关键词
unified reinforcement,configuration process,service demand,service quality assurance,vm resource budget,cloud computing,accurate service availability,near-optimal configuration,cloud dynamic,real-time autoconfiguration,autonomic cloud management,important service-oriented computing paradigm,reinforcement learning
AI 理解论文
溯源树
样例
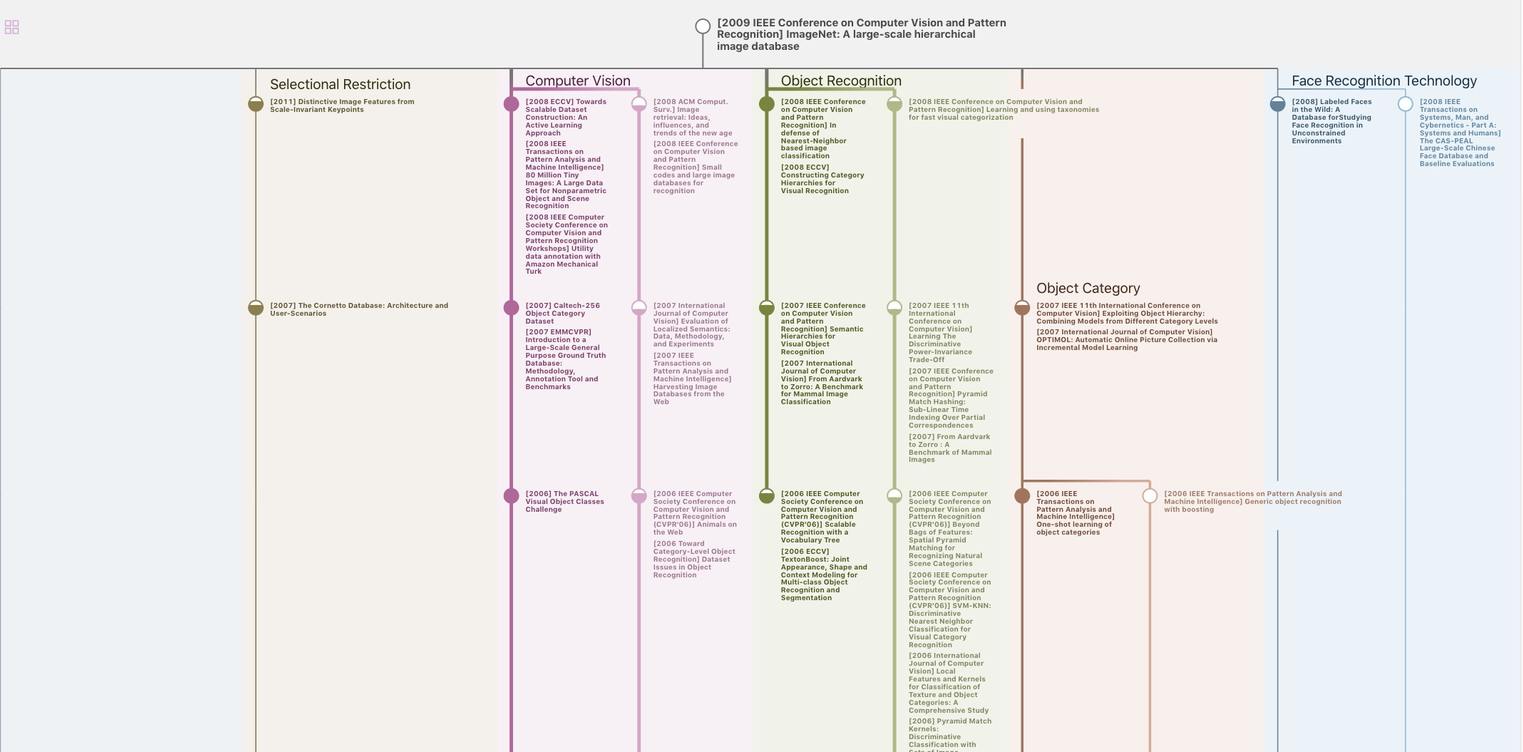
生成溯源树,研究论文发展脉络
Chat Paper
正在生成论文摘要