A Spectrum-Based Framework for Quantifying Randomness of Social Networks
IEEE Transactions on Knowledge and Data Engineering(2011)
摘要
Social networks tend to contain some amount of randomness and some amount of nonrandomness. The amount of randomness versus nonrandomness affects the properties of a social network. In this paper, we theoretically analyze graph randomness and present a framework which provides a series of nonrandomness measures at levels of edge, node, subgraph, and the overall graph. We show that graph nonrandomness can be obtained mathematically from the spectra of the adjacency matrix of the network. We derive the upper bound and lower bound of nonrandomness value of the overall graph. We investigate whether other graph spectra (such as Laplacian and normal spectra) could also be used to derive a nonrandomness framework. Our theoretical results showed that they are unlikely, if not impossible, to have a consistent framework to evaluate randomness. We also compare our proposed nonrandomness measures with some traditional measures such as modularity. Our theoretical and empirical studies show our proposed nonrandomness measures can characterize and capture graph randomness.
更多查看译文
关键词
normal spectra,nonrandomness measures,randomness measures,quantifying randomness,overall graph,graph spectrum,social network,social networks,nonrandomness framework,matrix algebra,consistent framework,adjacency matrix,nonrandomness measure,social networks.,graph spectra,laplacian spectra,proposed nonrandomness measure,randomness quantification,graph nonrandomness,graph theory,nonrandomness value,social networking (online),spectrum-based framework,graph randomness,random process,empirical study,random processes,spectrum,quantization,upper bound,lower bound,random measure
AI 理解论文
溯源树
样例
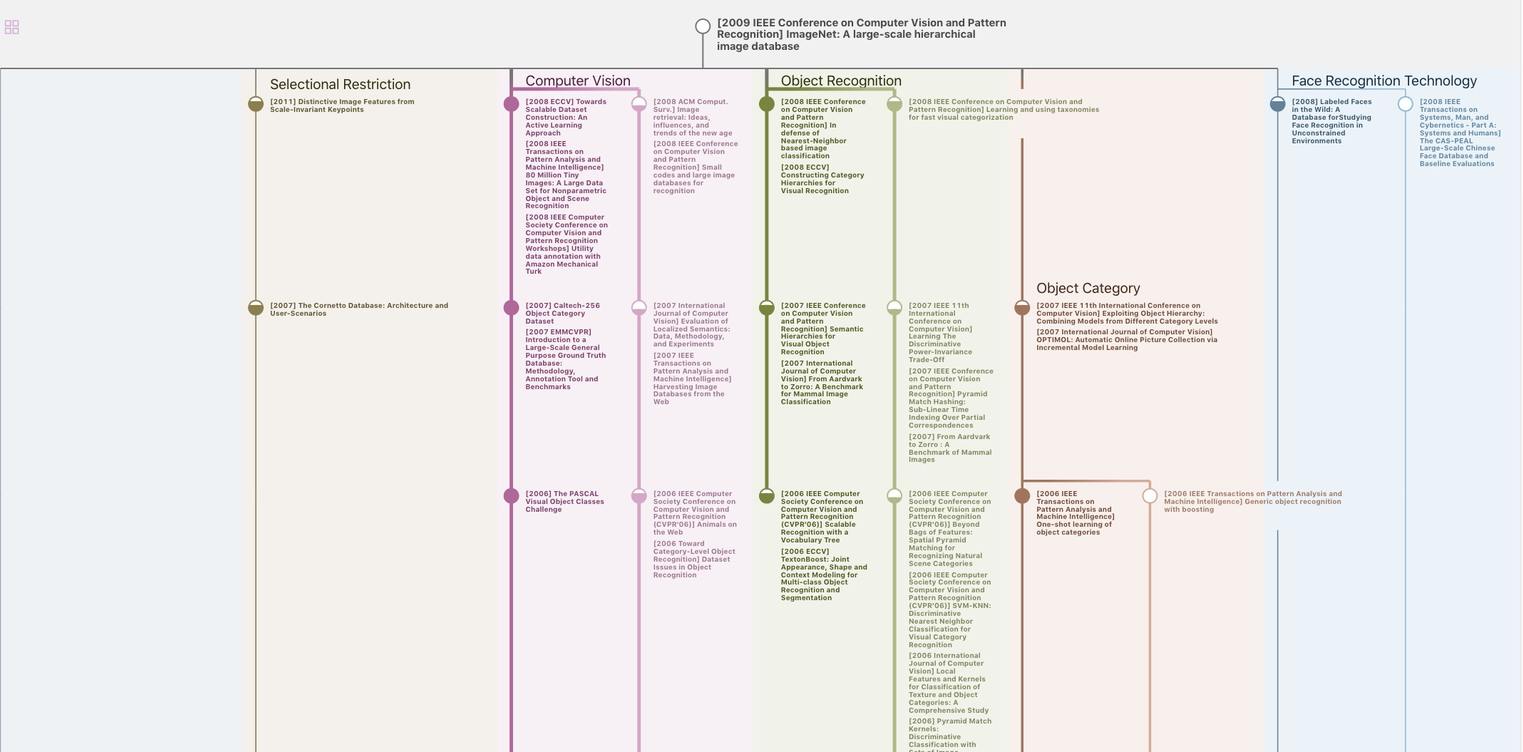
生成溯源树,研究论文发展脉络
Chat Paper
正在生成论文摘要