Sentence-based relevance flow analysis for high accuracy retrieval
JOURNAL OF THE AMERICAN SOCIETY FOR INFORMATION SCIENCE AND TECHNOLOGY(2011)
摘要
Traditional ranking models for information retrieval lack the ability to make a clear distinction between relevant and nonrelevant documents at top ranks if both have similar bag-of-words representations with regard to a user query. We aim to go beyond the bag-of-words approach to document ranking in a new perspective, by representing each document as a sequence of sentences. We begin with an assumption that relevant documents are distinguishable from nonrelevant ones by sequential patterns of relevance degrees of sentences to a query. We introduce the notion of relevance flow, which refers to a stream of sentence-query relevance within a document. We then present a framework to learn a function for ranking documents effectively based on various features extracted from their relevance flows and leverage the output to enhance existing retrieval models. We validate the effectiveness of our approach by performing a number of retrieval experiments on three standard test collections, each comprising a different type of document: news articles, medical references, and blog posts. Experimental results demonstrate that the proposed approach can improve the retrieval performance at the top ranks significantly as compared with the state-of-the-art retrieval models regardless of document type. © 2011 Wiley Periodicals, Inc.
更多查看译文
关键词
relevant document,ranking document,retrieval performance,nonrelevant document,sentence-based relevance flow analysis,existing retrieval model,retrieval experiment,document type,top rank,relevance flow,high accuracy retrieval,information retrieval lack
AI 理解论文
溯源树
样例
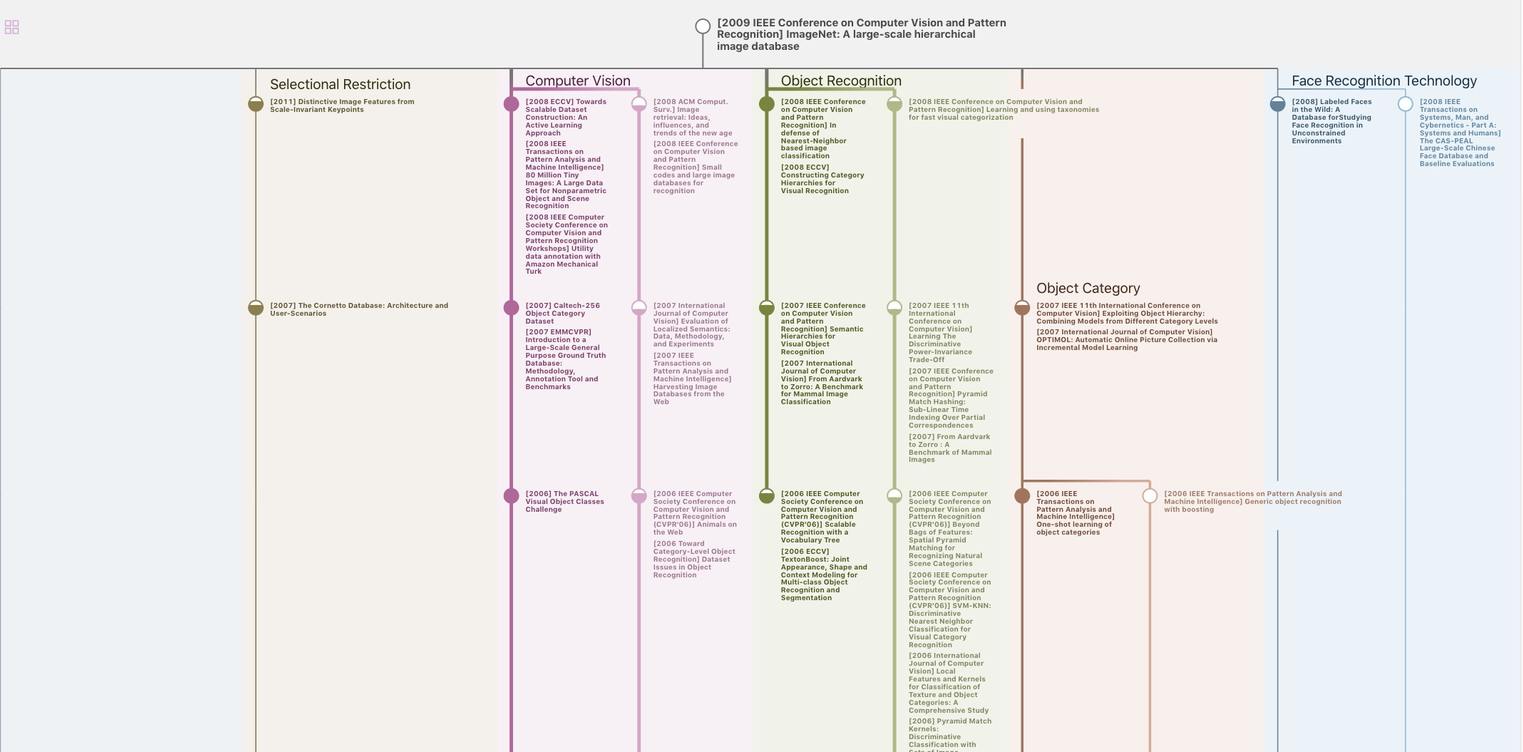
生成溯源树,研究论文发展脉络
Chat Paper
正在生成论文摘要