Large-Scale Customized Models for Advertisers
Data Mining Workshops(2010)
摘要
Performance advertisers want to maximize the return on their advertising spend. In the online advertising world, this means showing the ad only to those users most likely to convert i.e. buy a product or service. Existing ad targeting solutions such as context targeting and rule-based segment targeting primarily leverage marketing intuition to identify audience segments that would be likely to convert. Even the more sophisticated model-based approaches such as behavioral targeting identify audience segments interested in certain coarse-grained categories defined by the publisher. Advertisers are now able, through beaconing, to tell us exactly who their preferred customers are. Advertisers want to augment their existing advertising campaign with custom models that learn from the campaign and focus on attracting new users. Motivated by our experience with advertisers, we pose this problem within the context of ensemble learning. Building custom models for an existing ad campaign can be viewed as operations on an ensemble classifier: add, modify, or complement a classifier. An ideal new classifier should incrementally improve the ensemble and minimize overlap with any existing classifiers already in the ensemble–it should learn something new. With the proposed approach we are able to augment the advertising campaigns of several large advertisers at a large online advertising company.
更多查看译文
关键词
Internet,advertising data processing,knowledge based systems,learning (artificial intelligence),product customisation,advertisers,coarse grained categories,custom models,ensemble learning,marketing,model-based approach,online advertising company,online advertising world,rule-based segment
AI 理解论文
溯源树
样例
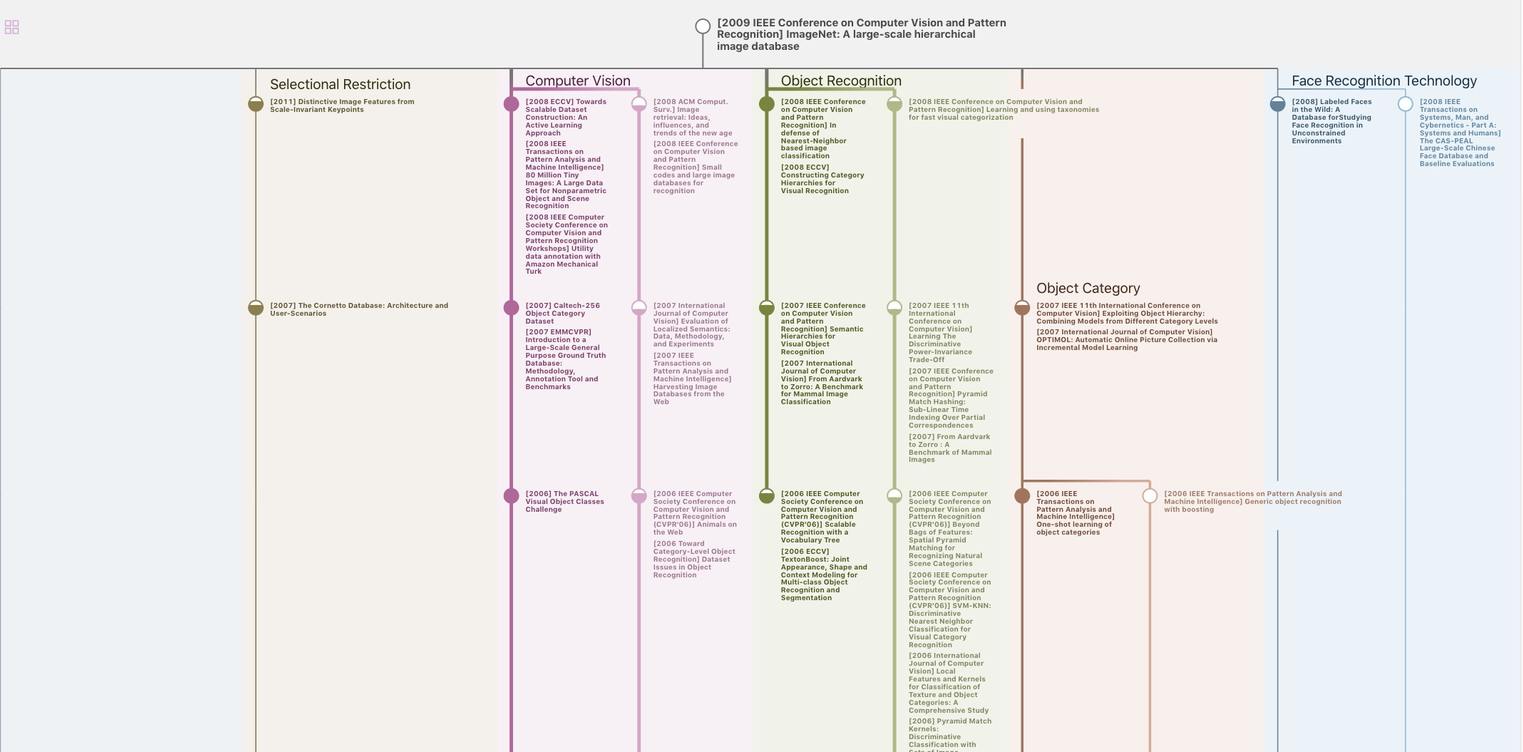
生成溯源树,研究论文发展脉络
Chat Paper
正在生成论文摘要