Distributed Classification on Peers with Variable Data Spaces and Distributions
Data Mining Workshops(2010)
摘要
The promise of distributed classification is to improve the classification accuracy of peers on their respective local data, using the knowledge of other peers in the distributed network. Though in reality, data across peers may be drastically different from each other (in the distribution of observations and/or the labels), current explorations implicitly assume that all learning agents receive data from the same distribution. We remove this simplifying assumption by allowing peers to draw from arbitrary data distributions and be based on arbitrary spaces, thus formalizing the general problem of distributed classification. We find that this problem is difficult because it does not admit state-of-the-art solutions in distributed classification. We also discuss the relation between the general problem and transfer learning, and show that transfer learning approaches cannot be trivially fitted to solve the problem. Finally, we present a list of open research problems in this challenging field.
更多查看译文
关键词
state-of-the-art solution,arbitrary data distribution,current exploration,general problem,variable data spaces,challenging field,open research problem,classification accuracy,respective local data,arbitrary space,computational modeling,support vector machines,transfer learning,distributed databases,accuracy,learning artificial intelligence,data models,silicon
AI 理解论文
溯源树
样例
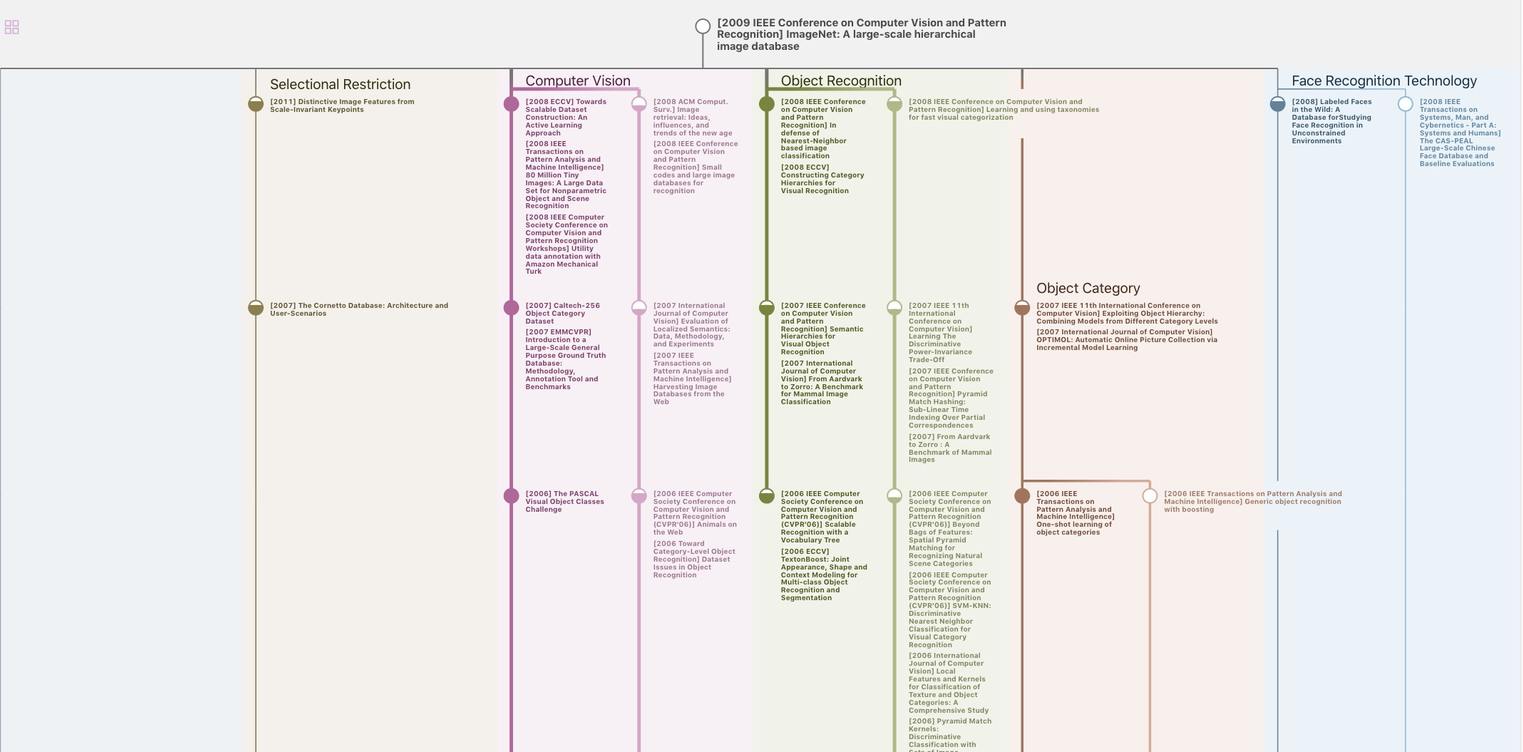
生成溯源树,研究论文发展脉络
Chat Paper
正在生成论文摘要