Comparing compact codebooks for visual categorization
Computer Vision and Image Understanding(2010)
摘要
In the face of current large-scale video libraries, the practical applicability of content-based indexing algorithms is constrained by their efficiency. This paper strives for efficient large-scale video indexing by comparing various visual-based concept categorization techniques. In visual categorization, the popular codebook model has shown excellent categorization performance. The codebook model represents continuous visual features by discrete prototypes predefined in a vocabulary. The vocabulary size has a major impact on categorization efficiency, where a more compact vocabulary is more efficient. However, smaller vocabularies typically score lower on classification performance than larger vocabularies. This paper compares four approaches to achieve a compact codebook vocabulary while retaining categorization performance. For these four methods, we investigate the trade-off between codebook compactness and categorization performance. We evaluate the methods on more than 200h of challenging video data with as many as 101 semantic concepts. The results allow us to create a taxonomy of the four methods based on their efficiency and categorization performance.
更多查看译文
关键词
codebook compactness,compact codebooks,efficient retrieval,categorization performance,benchmarking,concept categorization,excellent categorization performance,visual categorization,content analysis and indexing,video retrieval evaluation,compact codebook vocabulary,categorization efficiency,various visual-based concept categorization,compact vocabulary,concept categorization video retrieval evaluation efficient retrieval content analysis and indexing benchmarking,codebook model,classification performance,indexation,content analysis
AI 理解论文
溯源树
样例
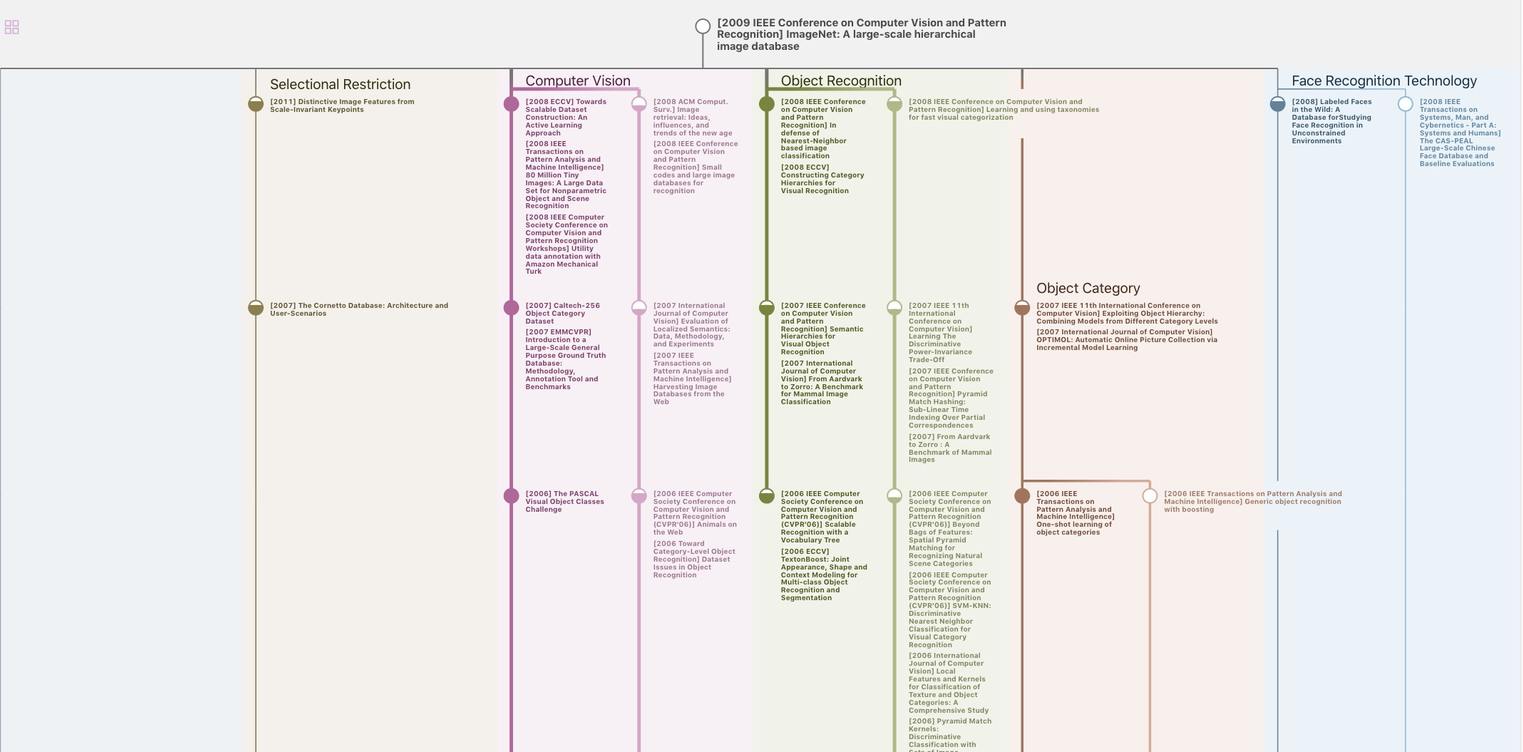
生成溯源树,研究论文发展脉络
Chat Paper
正在生成论文摘要