Ontology mapping: as a binary classification problem
SKG '08 Proceedings of the 2008 Fourth International Conference on Semantics, Knowledge and Grid(2011)
摘要
Ontology mapping (OM) seeks to find semantic correspondences between similar elements of different ontologies. OM is critical to achieve semantic interoperability in the World Wide Web. To solve the OM problem, this article proposes a non-instance learning-based approach that transforms the OM problem into a binary classification problem and utilizes machine learning techniques as a solution. Same as other machine learning-based approaches, a number of features (i.e. linguistic, structural, and web features) are generated for each mapping candidate. However, in contrast to other learning-based mapping approaches, the features proposed in our approach are generic and do not rely on the existence and sufficiency of instances. Therefore, our approach can be generalized to different domains without extra training efforts. To evaluate our approach, two experiments (i.e. within-task vs cross-task) are implemented and the SVM (support vector machine) algorithm is applied. Experimental results show that our non-instance learning-based OM approach performs well on most of OAEI benchmark tests when training and testing on the same mapping task; and the results of approach vary according to the likelihood of training data and testing data when training and testing on different mapping tasks. Copyright © 2010 John Wiley & Sons, Ltd.
更多查看译文
关键词
mapping task,ontology mapping,extra training effort,mapping candidate,binary classification problem,non-instance learning-based approach,OM approach,different mapping task,learning-based mapping approach,machine learning-based approach,OM problem
AI 理解论文
溯源树
样例
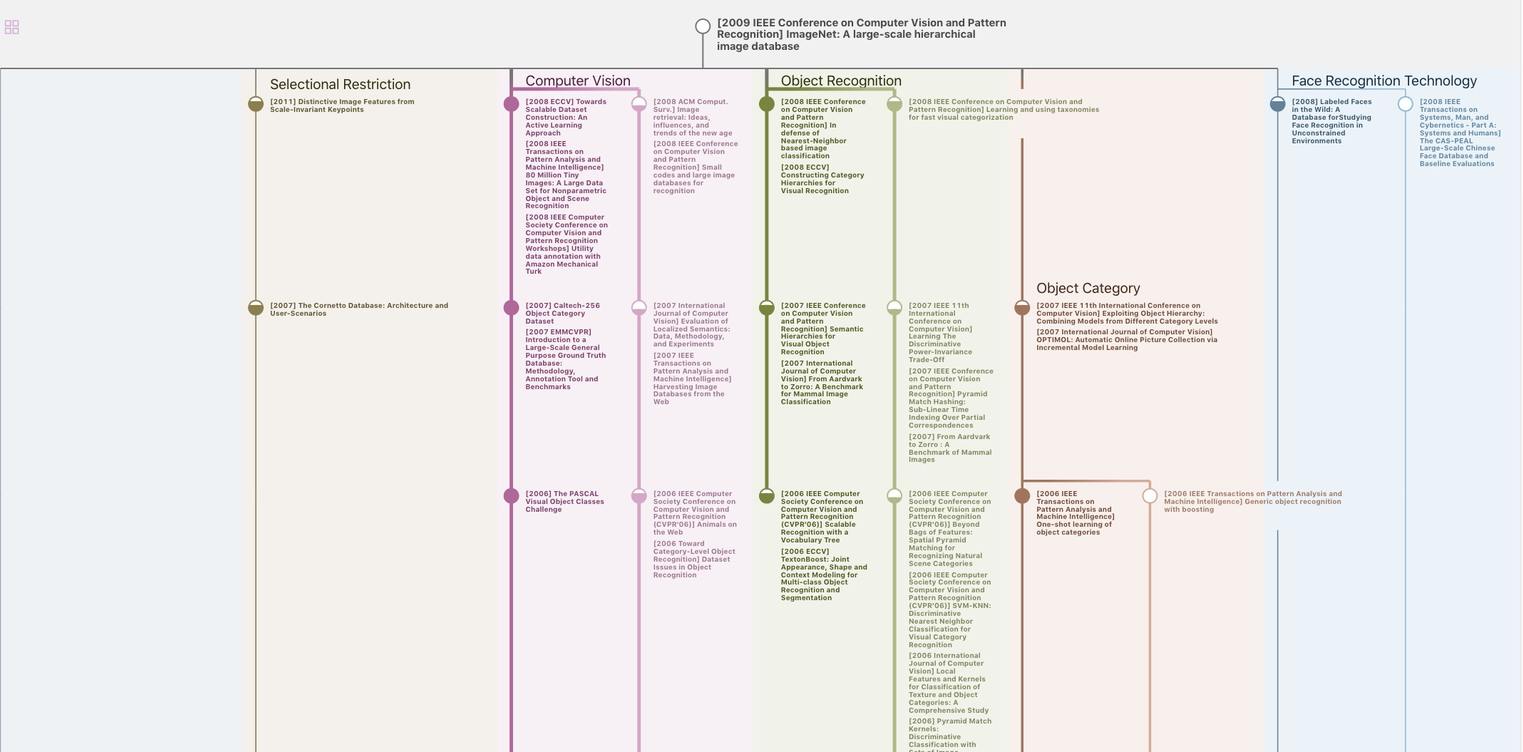
生成溯源树,研究论文发展脉络
Chat Paper
正在生成论文摘要