Learning all optimal policies with multiple criteria
ICML(2008)
摘要
We describe an algorithm for learning in the presence of multiple criteria. Our technique generalizes previous approaches in that it can learn optimal policies for all linear preference assignments over the multiple reward criteria at once. The algorithm can be viewed as an extension to standard reinforcement learning for MDPs where instead of repeatedly backing up maximal expected rewards, we back up the set of expected rewards that are maximal for some set of linear preferences (given by a weight vector, w). We present the algorithm along with a proof of correctness showing that our solution gives the optimal policy for any linear preference function. The solution reduces to the standard value iteration algorithm for a specific weight vector, w.
更多查看译文
关键词
linear preference assignment,standard value iteration algorithm,linear preference function,expected reward,specific weight vector,linear preference,multiple criterion,optimal policy,standard reinforcement,multiple reward criterion,value iteration,reinforcement learning
AI 理解论文
溯源树
样例
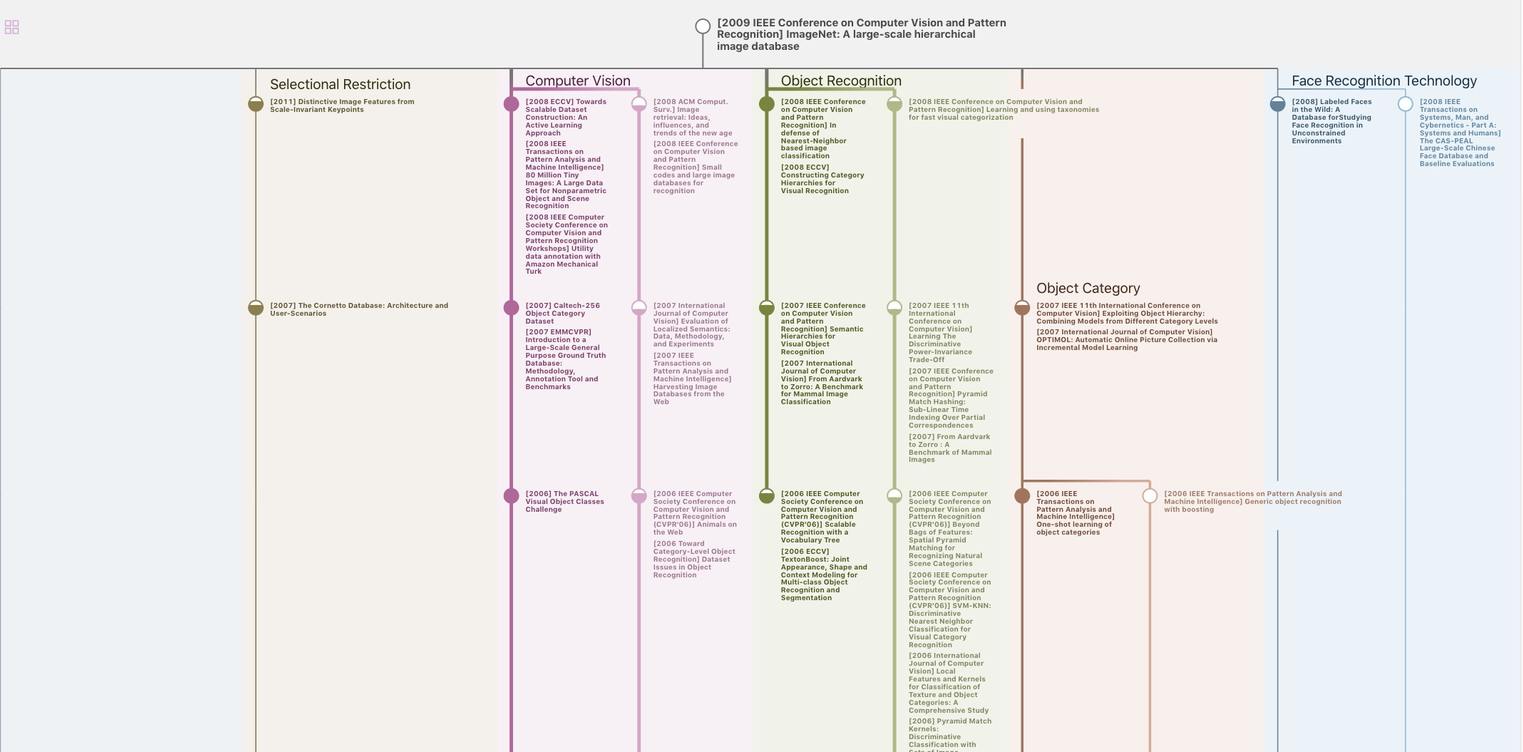
生成溯源树,研究论文发展脉络
Chat Paper
正在生成论文摘要