Using One-Class and Two-Class SVMs for Multiclass Image Annotation
IEEE Transactions on Knowledge and Data Engineering(2005)
摘要
We propose using one-class, two-class, and multiclass SVMs to annotate images for supporting keyword retrieval of images. Providing automatic annotation requires an accurate mapping of images' low-level perceptual features (e.g., color and texture) to some high-level semantic labels (e.g., landscape, architecture, and animals). Much work has been performed in this area; however, there is a lack of ability to assess the quality of annotation. In this paper, we propose a confidence-based dynamic ensemble (CDE), which employs a three-level classification scheme. At the base-level, CDE uses one-class Support Vector Machines (SVMs) to characterize a confidence factor for ascertaining the correctness of an annotation (or a class prediction) made by a binary SVM classifier. The confidence factor is then propagated to the multiclass classifiers at subsequent levels. CDE uses the confidence factor to make dynamic adjustments to its member classifiers so as to improve class-prediction accuracy, to accommodate new semantics, and to assist in the discovery of useful low-level features. Our empirical studies on a large real-world data set demonstrate CDE to be very effective.
更多查看译文
关键词
empirical study,support vector machine,landscape architecture,artificial intelligent,learning artificial intelligence,image classification,data mining,indexing terms,image annotation,support vector machines,image retrieval,pattern recognition,feature extraction,artificial intelligence
AI 理解论文
溯源树
样例
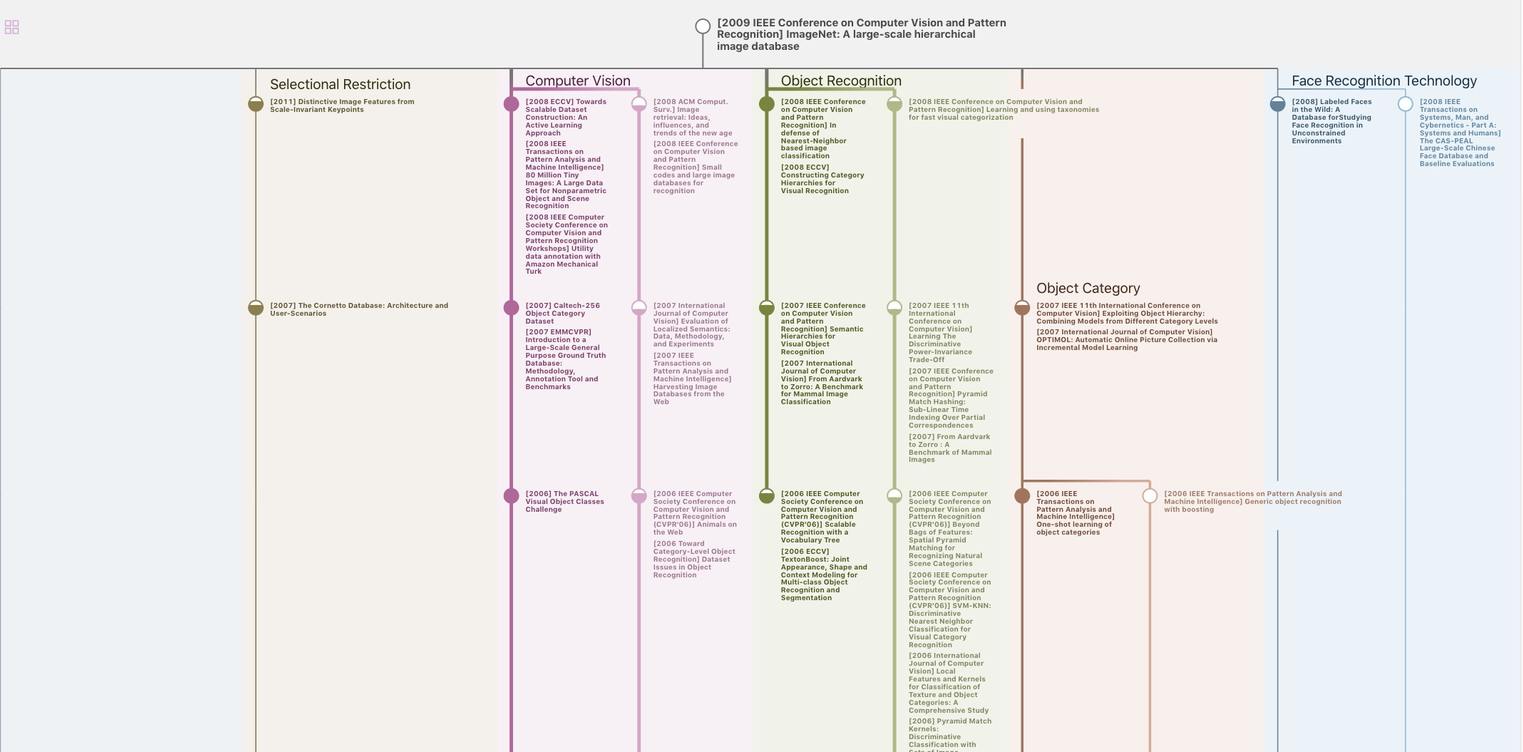
生成溯源树,研究论文发展脉络
Chat Paper
正在生成论文摘要