Lessons and Challenges from Mining Retail E-Commerce Data
Machine Learning(2004)
摘要
The architecture of Blue Martini Software's e-commerce suite has supported data collection, data transformation, and data mining since its inception. With clickstreams being collected at the application-server layer, high-level events being logged, and data automatically transformed into a data warehouse using meta-data, common problems plaguing data mining using weblogs (e.g., sessionization and conflating multi-sourced data) were obviated, thus allowing us to concentrate on actual data mining goals. The paper briefly reviews the architecture and discusses many lessons learned over the last four years and the challenges that still need to be addressed. The lessons and challenges are presented across two dimensions: business-level vs. technical, and throughout the data mining lifecycle stages of data collection, data warehouse construction, business intelligence, and deployment. The lessons and challenges are also widely applicable to data mining domains outside retail e-commerce.
更多查看译文
关键词
data mining,data analysis,business intelligence,web analytics,web mining,OLAP,visualization,reporting,data transformations,retail,e-commerce,Simpson's paradox,sessionization,bot detection,clickstreams,application server,web logs,data cleansing,hierarchical attributes,business reporting,data warehousing
AI 理解论文
溯源树
样例
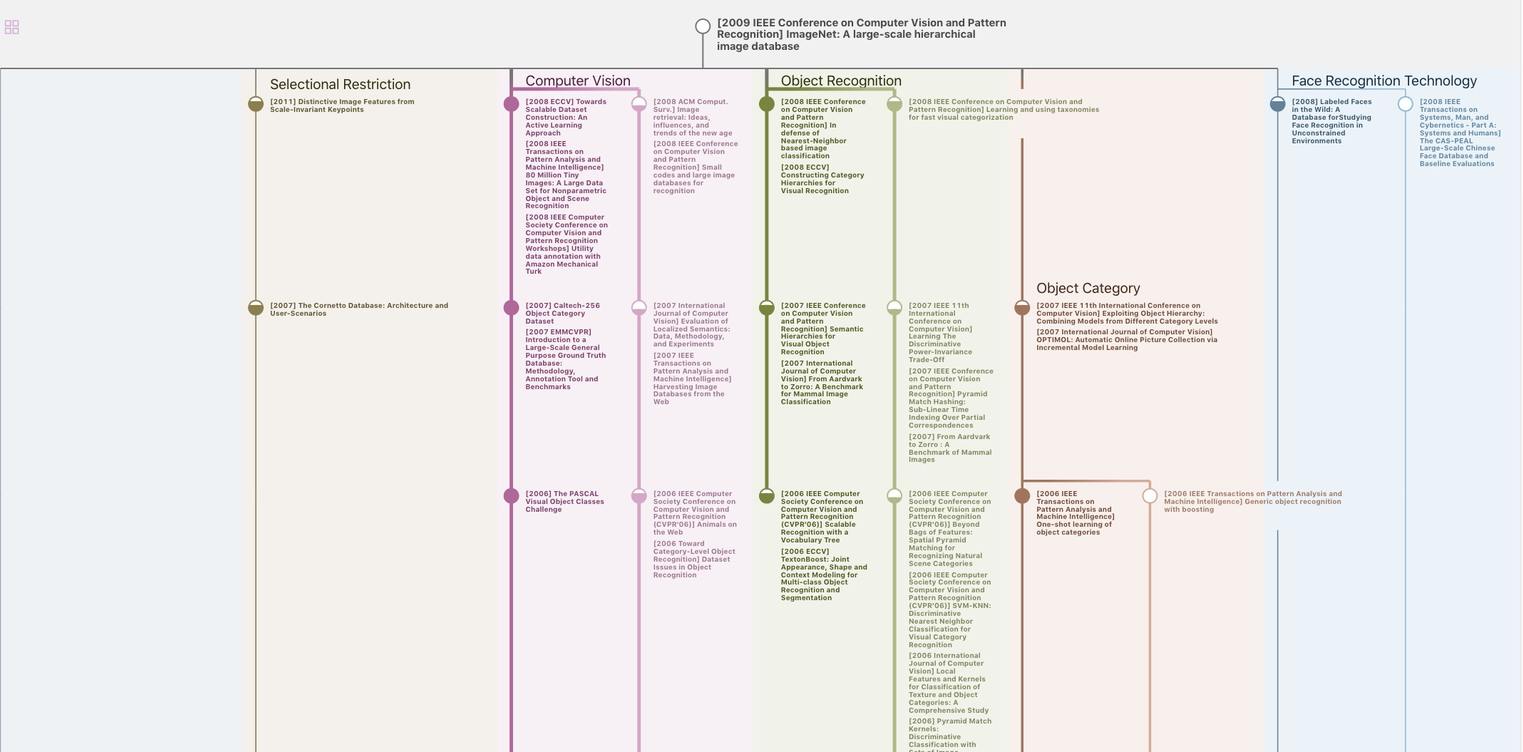
生成溯源树,研究论文发展脉络
Chat Paper
正在生成论文摘要